Zircoo S/4 Hana Kafka Connector
The S/4 Hana Connector improves system performance by linking SAP S/4 Hana with other applications.
S/4 Hana Connector
Abstract
While a SAP ERP system may struggle to retain the entire history with every individual change due to the sheer volume of data, the potential with the right technical solution is boundless.
By consistently transferring change data from S/4 Hana to Apache Kafka, the ERP system becomes the real-time business backbone, with Kafka serving as the real-time change log, offering unprecedented capabilities.
Use Cases
All changes from the ERP system are streamed into the target.
All changes of remote systems are streamed into the ERP system.
All changes since the last delta are and loaded into SAP BW.
All data comes in two flavors, the original data and a version with cleansed data. Consumers can pick either.
As the cleansing result is attached to the data, reporting on the data is easy. “Find all records that violated Rule #2”
A consumer of the cleansed data can trigger alerts to notify users immediately of severe issues.
In today’s world the ERP system provides data to multiple connected applications. If the ERP system has to provide the data just once and all downstream systems pick it up from there, the ERP system is freed up from sending the same changes to multiple systems.
Storyline
Every change in the ERP system is immediately posted to Apache Kafka. Consumers can then either listen for changes with sub-second latency for real-time processing or read the changes at their convenience.
Apache Kafka functions similarly to a database transaction log but is optimized for Big Data scenarios, making it ideal for storing massive amounts of intermediate changes.
Careful attention has been paid to maintaining data consistency within Kafka.
Services can now listen to the changed data and enhance its quality. Examples include:
- Validation service: Applies rules to each incoming record, attaching information indicating if the record is good, bad, or a warning, along with details of the rules tested and their results.
- Cleansing service: Standardizes incoming data, such as standardizing spelling variations for gender.
- Enrichment service: Derives new information from incoming data, like deriving sales region from country codes.
- Profiling service: Updates current statistics as data streams in to identify patterns, such as increasing incomplete data from a specific source system.
- Alerting service: Proactively sends alerts, such as sending an email if a mandatory field from a source is missing.
- ML service for customer categorization: Utilizes machine learning to categorize customers based on data attributes.
How it works
A Producer is a web application connected to a source system and sending changes to Apache Kafka. The producers identifies the changes in the source system, assembles the business object and sends the changes.
Consumers read the data from Apache Kafka and write it into the target system. Consumers are in full control of what they want to read. They can read the data since the last read (batch delta), they can listen to changes (realtime streaming), they can read the transaction log from any point in time.
Services are implemented as Kafka KStreams and perform inflight operations on the data. A Rules service for example would listen to new data, run the various tests, adds the test results to the record and puts the data into Kafka again.
Is the backend to choreograph the data between producer, consumer and services. The data is organized in topics – these are the change data queues – and schemas, the business objects. Within a topic the data is processed strictly in order, so for a perfect transactional guarantee all data has to go through a single topic at the costs of less parallel processing.
Architecture
At the core of the solution lies Apache Kafka, serving as the backend for data production, consumption, and microservice attachment.
Kafka’s schema registry maintains the logical data model of all data structures, offering flexibility akin to Big Data paradigms, including schema evolution, extension concepts, and compatibility assurance.
A Metadata store within Kafka tracks the lineage of services and the current landscape.
While producers and consumers can interact with Kafka directly via the native TCP protocol, data streaming is typically facilitated via HTTPS to maintain network security.
Kafka supports streaming transformations to implement various services, with consumers having the freedom to choose the data they consume and when, assuming they have the required permissions.
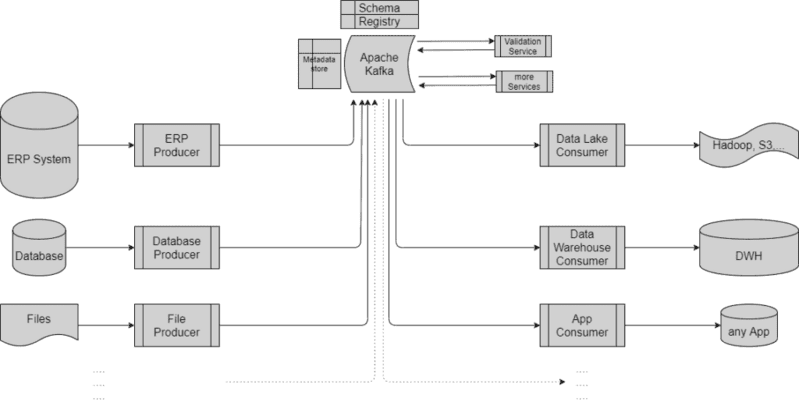
Data Model
Kafka itself can deal with any kind of payload. This flexibility is nice but data integration without even knowing the structure is, well, difficult.
Therefore Kafka is backed by a schema registry where all known schemas are available, including additional metadata, and producers have the task to produce the data according to the structure and the rules of the schemas. Having said that, producers can create or extend schemas automatically.
On the root level, each schema has additional fields about record origin, time of change, type of change.
On the root level is a structure to store transformation results. Every producer and service can add information. As a result the consumer knows what transformations a record did undergo. For example a FileProducer can explain that one line ended premature and categorize that fact as a warning. A validation service can complain that a field was not set although it should have been.
Every record level has an Extension array. This is an opportunity to store any data as key-value pairs without the need to modify the data model at all.
For example the logical data model defines a field GENDER as a string but the source system has a number instead where “1” stands for “female”. Could be interesting to add the original number for auditing reasons. Hence the producer adds the key-value pair “Gender original value” = 1 into the Extension array.
Although a relational schema is possible, as one producer is generating the data for many consumers and services, the suggestion is to go more towards sending business objects.
In the left side example the data is stored in two tables in the source, the order header and the line items table. But instead of sending change information on each of the two tables, produce the entire sales order, with the line items being a sub-array of the order. Yes, that requires a join but better join once than every consumer has to perform the join individually.